The term modal in mathematics refers to the value that appears most frequently in a dataset. Alongside the mean and median, it is a key measure of central tendency, helping to summarize data and identify patterns or trends. The mode is particularly useful in analyzing categorical and numerical data, offering insights into common occurrences.
This blog delves into the mode concept, its significance in data analysis, and the different types of modal values. It also provides a step-by-step guide on how to calculate the mode, complete with practical examples and real-world applications.
Understanding the Modal in Maths
The mode is one of mathematics’s simplest yet most insightful measures of central tendency. Defined as the value that occurs most frequently in a dataset, the mode is unique in its focus on frequency rather than averages or positions. Unlike the mean, which calculates the arithmetic average, or the median, which identifies the middle value in an ordered dataset, the mode highlights the most common value, making it particularly effective for analyzing trends and preferences.
The mode is critical in both numerical and categorical data. For numerical datasets, it identifies repeated values, offering insights into patterns within the data. For instance, in a dataset of daily temperatures, the mode could reveal the most frequent temperature, providing a sense of typical weather conditions. In categorical data, such as survey responses, the mode shows the most popular choice or opinion, helping researchers understand trends and preferences.
The mode’s importance lies in its simplicity and applicability. It’s especially useful when analyzing datasets where frequency matters, such as identifying the most commonly purchased product in a store or determining the most frequent customer complaint in a service industry. In education, teachers use the mode to assess the most common grades among students, helping them tailor their teaching strategies to meet the class’s needs. The mode’s ability to summarize large datasets into meaningful insights makes it a versatile and valuable tool in data analysis.
Types of Modal Data
Datasets can exhibit different modal characteristics depending on how values are distributed. Understanding these variations is essential for interpreting data accurately.
Unimodal Data
Unimodal datasets have a single mode, meaning one value occurs more frequently than any other. For example, in the dataset [3, 5, 7, 7, 9], the mode is 7 because it appears twice, more than any other number. Unimodal datasets are the most straightforward to analyze, as they highlight the most common value.
Bimodal Data
Bimodal datasets have two modes, with two values occurring at the highest frequency. For instance, in the dataset [2, 4, 4, 6, 8, 8], the modes are 4 and 8, as both appear twice. Bimodal distributions often indicate two dominant groups or trends within the data. For example, in a survey of favorite activities among students, two modes might represent equal preferences for sports and music.
Multimodal Data
Multimodal datasets contain more than two modes, indicating multiple values with the same highest frequency. For example, in the dataset [1, 2, 2, 3, 3, 4, 4], the modes are 2, 3, and 4. Multimodal datasets are less common but can arise in diverse or segmented populations. For instance, in a city with a multicultural population, multiple modes might represent distinct groups with unique preferences.
No Mode
In some datasets, all values occur with equal frequency, resulting in no mode. For example, in the dataset [1, 2, 3, 4, 5], each value appears only once. These datasets often lack patterns or trends, making other measures of central tendency, such as the mean or median, more suitable for analysis.
Recognizing the type of modal data is crucial for selecting appropriate analytical methods and drawing accurate conclusions.
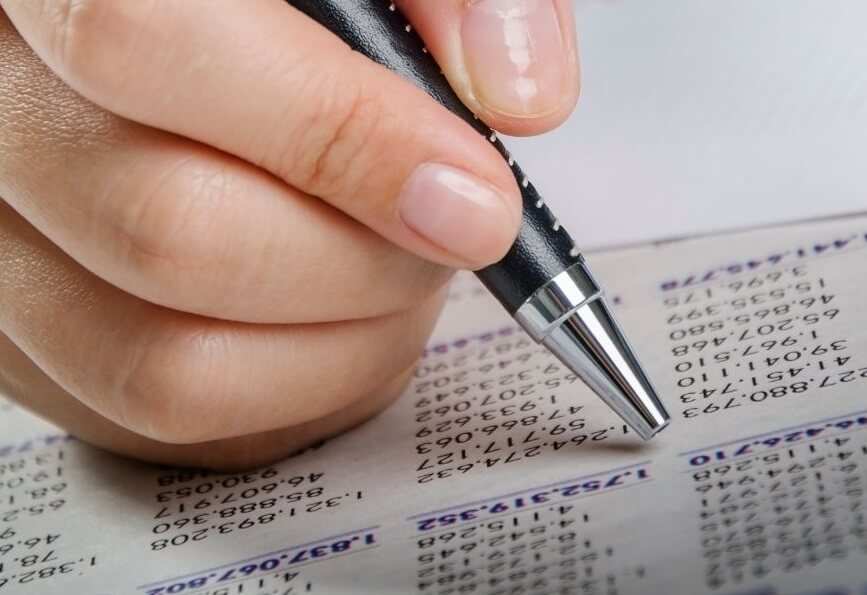
How to Calculate the Mode
The process of calculating the mode varies depending on whether the data is numerical, categorical, or grouped. Each type requires a tailored approach to identify the most frequently occurring value.
For Numerical Data
To calculate the mode in a numerical dataset, begin by organizing the data in ascending order. Then, count the frequency of each value. The mode is the value that appears most frequently.
Example: Consider the dataset [2, 3, 3, 5, 6, 6, 6, 7].
- Arrange the data: [2, 3, 3, 5, 6, 6, 6, 7].
- Count frequencies: 2 → 1, 3 → 2, 5 → 1, 6 → 3, 7 → 1.
- The mode is 6, as it appears three times, more than any other value.
For Categorical Data
In categorical data, the mode identifies the category or label that occurs most frequently. This is particularly useful in survey data, where responses are non-numerical.
Example: Consider a survey asking for favorite colors: [“red,” “blue,” “red,” “green,” “blue,” “red”].
- Count frequencies: red → 3, blue → 2, green → 1.
- The mode is “red,” as it is the most frequently mentioned response.
For Grouped Data
Grouped data, often presented in frequency tables, requires identifying the modal class—the interval with the highest frequency. Calculating the mode for grouped data may also involve interpolation for greater precision.
Example: Consider the frequency table:
- Interval: 0–10, Frequency: 5
- Interval: 10–20, Frequency: 8
- Interval: 20–30, Frequency: 12
- Interval: 30–40, Frequency: 7
The modal class is 20–30, as it has the highest frequency (12). For detailed calculations, interpolation formulas can estimate the exact mode within the class.
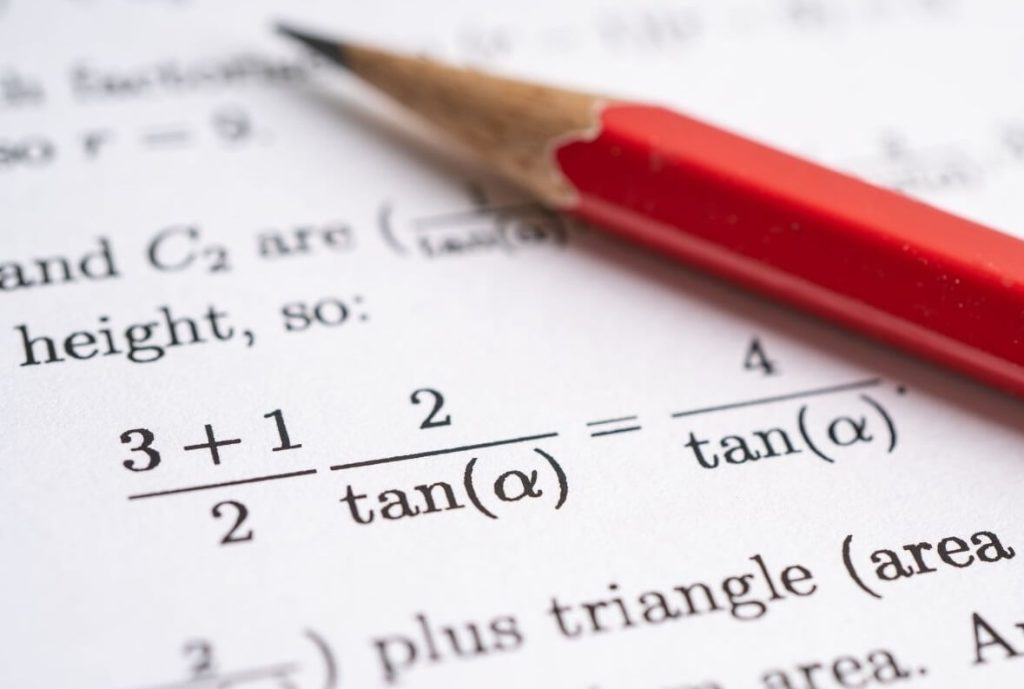
Applications of the Modal Value
The mode is a versatile tool with applications in various fields, providing insights into trends, preferences, and patterns.
In Business
Businesses frequently use the mode to understand consumer preferences and optimize operations. For example, a clothing retailer might identify the most popular size or color of a product to adjust inventory and meet customer demand. Similarly, restaurants use the mode to determine the most frequently ordered dishes, helping them streamline menus and manage supplies.
In Education
In education, the mode helps teachers and administrators analyze student performance. By identifying the most common grades, educators can assess overall class performance and design interventions to address gaps. For example, if a mode of 70% is identified in a dataset of exam scores, it highlights the most frequently achieved grade, guiding teachers to focus on helping students improve beyond this level.
In Healthcare
Healthcare professionals use the mode to identify common symptoms or conditions in patient populations. For example, a clinic analyzing patient data might find that fever is the most frequently reported symptom during flu season. This insight allows healthcare providers to allocate resources and plan interventions effectively.
In Everyday Life
The mode also plays a role in everyday decisions. For instance, car manufacturers analyze the most popular colors among buyers to determine production priorities. Similarly, streaming platforms use the mode to recommend the most-watched genres or shows to their users, enhancing customer satisfaction.
Comparing Mode with Other Measures of Central Tendency
When discussing measures of central tendency, the mode is often compared to the mean and median, as each serves a distinct purpose in data analysis.
Mode vs. Mean
The mode and mean approach data analysis from different perspectives. The mode focuses on frequency, identifying the most common value in a dataset, while the mean calculates the arithmetic average by dividing the sum of all values by the number of observations. For instance, in the dataset [2, 3, 3, 5, 6], the mode is 3 because it appears most frequently, while the mean is (2 + 3 + 3 + 5 + 6) ÷ 5 = 3.8.
The mode is often more useful than the mean in scenarios where frequency matters. For example, a retailer analyzing customer shoe sizes will find the mode more informative, as it identifies the most commonly purchased size. In contrast, the mean is better suited for datasets with continuous numerical values and even distributions, such as calculating average temperatures over a week. However, the mean can be skewed by extreme values or outliers, making the mode a more reliable indicator in some contexts.
Mode vs. Median
The mode and median also differ in their approach to analyzing data. While the mode identifies the most frequent value, the median determines the middle value in an ordered dataset. For example, in the dataset [2, 3, 3, 5, 6, 8], the mode is 3, while the median is the average of the two middle values (3 and 5), which is 4.
The median’s positional nature makes it more robust in the presence of outliers. For instance, in a dataset of house prices [£100,000, £150,000, £200,000, £250,000, £10,000,000], the mean would be heavily skewed by the outlier (£10,000,000), while the median (£200,000) provides a more accurate representation of typical prices. However, the mode could reveal the most frequently occurring price range, offering additional insights. Combining the mode and median in such analyses often provides a fuller picture, especially when interpreting skewed or diverse datasets.
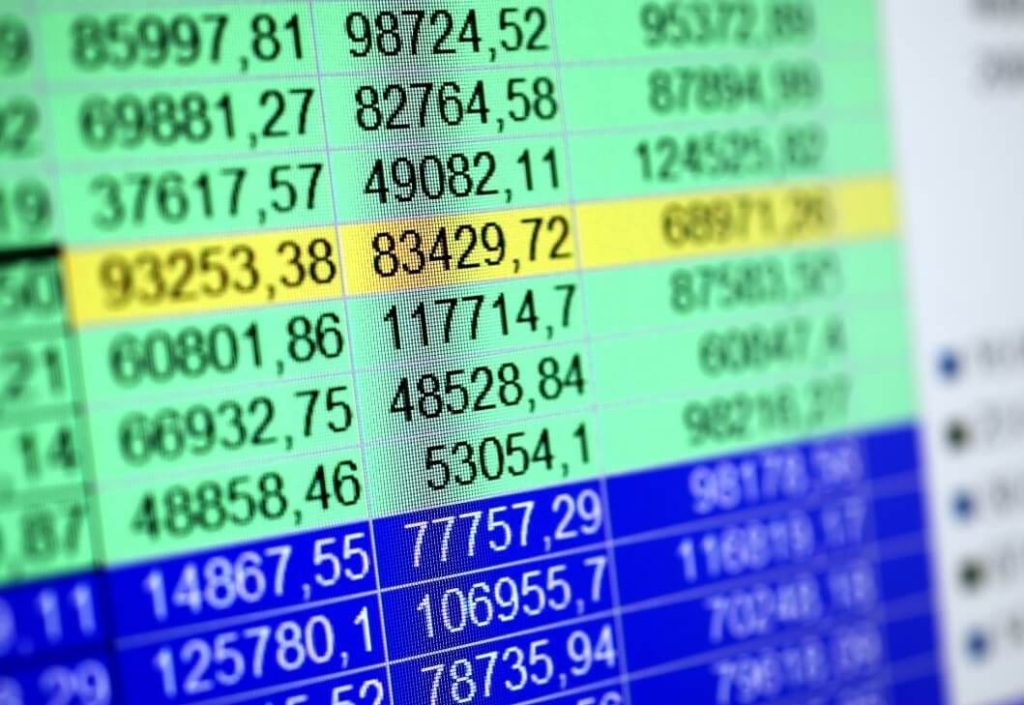
Challenges and Limitations of the Mode
While the mode is a valuable measure of central tendency, it is not without its challenges and limitations.
Ambiguity in Multimodal Data
One of the key challenges in using the mode arises in multimodal datasets, where multiple values occur with the same highest frequency. For example, in the dataset [2, 2, 4, 4, 6, 6], the modes are 2, 4, and 6. While identifying multiple modes provides information about the dataset’s diversity, it can complicate interpretation. Analysts must decide whether to consider all modes equally or prioritize specific ones based on context.
Limited Use in Continuous Data
The mode is less informative for continuous datasets where values do not repeat frequently or at all. For instance, in a dataset of precise measurements like [1.23, 1.45, 1.67, 1.89, 2.01], there may be no clear mode, as all values are unique. In such cases, the mean or median is often more appropriate for summarizing the data.
Over-reliance on Mode
Another limitation of the mode is its potential to misrepresent central tendency in certain datasets. For example, in [2, 2, 2, 8, 10, 12], the mode is 2, but it does not accurately reflect the overall distribution of values. Over-reliance on the mode in such cases could lead to skewed interpretations, emphasizing the need to combine it with other measures like the mean or median.
Recognizing these limitations is crucial for using the mode effectively and ensuring accurate data analysis.
Practical Examples of Calculating the Mode
Example 1: Favorite Ice Cream Flavors
Imagine a survey where participants are asked their favorite ice cream flavor, resulting in the dataset [“Vanilla,” “Chocolate,” “Vanilla,” “Strawberry,” “Vanilla,” “Chocolate”]. To calculate the mode:
- Count the occurrences of each flavor: Vanilla (3), Chocolate (2), Strawberry (1).
- The mode is “Vanilla,” as it is the most frequently mentioned flavor. This insight can help ice cream shops prioritize stocking vanilla to meet customer preferences.
Example 2: Exam Scores
Consider a dataset of student exam scores: [70, 75, 80, 80, 85, 90, 90, 90, 95]. To identify the mode:
- Count the frequency of each score: 70 (1), 75 (1), 80 (2), 85 (1), 90 (3), 95 (1).
- The mode is 90, as it appears most frequently. This information could help teachers identify a performance benchmark for the class.
Example 3: Customer Product Choices
In a retail store, a frequency table of sold T-shirt sizes might look like this:
- Small: 10 units.
- Medium: 25 units.
- Large: 15 units.
- Extra Large: 5 units.
The mode is “Medium,” as it has the highest frequency (25 units sold). Retailers can use this information to stock more medium-sized T-shirts, ensuring they meet customer demand effectively.
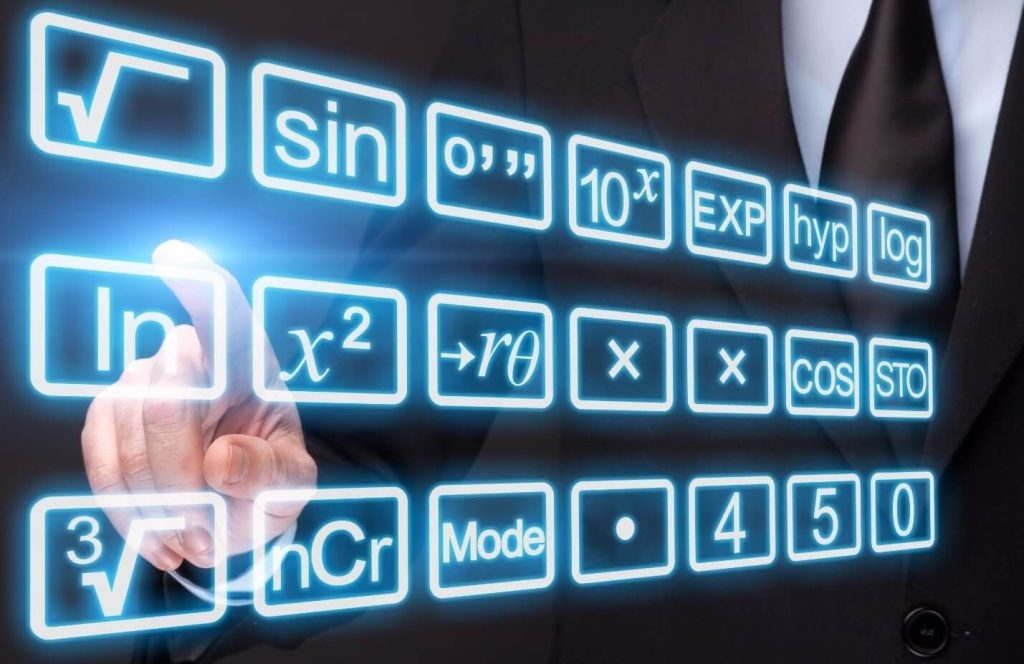
Extending Modal Concepts
The concept of the mode can be extended and adapted for more complex scenarios, such as weighted datasets, grouped data, and probability analysis.
Weighted Mode
In some cases, data values are associated with weights, and the weighted mode is the value with the highest weighted frequency. For example, if a dataset represents sales of different products and their respective profit margins, the weighted mode identifies the product that contributes the most profit overall. This is particularly useful in business decision-making.
Modal Class in Statistics
In grouped data, the mode refers to the modal class, which is the class interval with the highest frequency. For example, in a frequency distribution of student heights:
- 150–160 cm: 5 students.
- 160–170 cm: 10 students.
- 170–180 cm: 7 students.
The modal class is 160–170 cm, as it has the highest frequency. Modal class analysis is widely used in statistics to interpret continuous data and identify dominant trends.
Connection to Probability
The mode is also closely related to probability, as it represents the most likely outcome in a dataset. In probability distributions, the mode is the peak point, indicating the value with the highest likelihood of occurrence. For example, in a dice-rolling experiment, the mode of outcomes (e.g., most frequently rolled number) helps predict future results, aiding decision-making in games and simulations.
Conclusion
The mode, the most frequently occurring value in a dataset, is a key measure of central tendency. Its versatility spans across various types—unimodal, bimodal, and multimodal—and its calculation is straightforward, whether for numerical, categorical, or grouped data.
The mode plays a crucial role in data analysis, helping to identify patterns and trends in fields like business, education, and healthcare. By practicing its calculation and interpretation, you can apply this powerful tool to real-world scenarios, gaining valuable insights from data with ease.